Adaptive scheduling in Spark
Author(s)
Mahajan, Rohan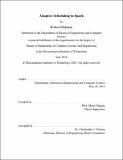
DownloadFull printable version (414.4Kb)
Other Contributors
Massachusetts Institute of Technology. Department of Electrical Engineering and Computer Science.
Advisor
Matei Zaharia.
Terms of use
Metadata
Show full item recordAbstract
Because most data processing systems are distributed in nature, data must be transferred between machines. Currently, Spark, a prominent such system, predetermines the strategies for shuffling this data, but in certain situations, different shuffle strategies would improve performance. We add functionality to track metrics about the data during the job and appropriately adapt the shuffle strategy. We show improvements in ShuffledRDD performance, joins using Spark's RDD interface, and joins in Spark SQL.
Description
Thesis: M. Eng., Massachusetts Institute of Technology, Department of Electrical Engineering and Computer Science, 2016. This electronic version was submitted by the student author. The certified thesis is available in the Institute Archives and Special Collections. Cataloged from student-submitted PDF version of thesis. Includes bibliographical references (page 33).
Date issued
2016Department
Massachusetts Institute of Technology. Department of Electrical Engineering and Computer SciencePublisher
Massachusetts Institute of Technology
Keywords
Electrical Engineering and Computer Science.