On Generalization Bounds for Neural Networks with Low Rank Layers
Author(s)
Pinto, Andrea; Rangamani, Akshay; Poggio, Tomaso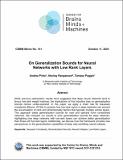
DownloadCBMM-Memo-151.pdf (697.3Kb)
Metadata
Show full item recordAbstract
While previous optimization results have suggested that deep neural networks tend to favour low-rank weight matrices, the implications of this inductive bias on generalization bounds remain under-explored. In this paper, we apply a chain rule for Gaussian complexity (Maurer, 2016a) to analyze how low-rank layers in deep networks can prevent the accumulation of rank and dimensionality factors that typically multiply across layers. This approach yields generalization bounds for rank and spectral norm constrained networks. We compare our results to prior generalization bounds for deep networks, highlighting how deep networks with low-rank layers can achieve better generalization than those with full-rank layers. Additionally, we discuss how this framework provides new perspectives on the generalization capabilities of deep nets exhibiting neural collapse.
Date issued
2024-10-11Publisher
Center for Brains, Minds and Machines (CBMM)
Series/Report no.
CBMM Memo;151
Keywords
Gaussian Complexity, Generalization Bounds, Low Rank Layers, Neural Collapse