PCA as a defense against some adversaries
Author(s)
Aparne, Gupta; Banburski, Andrzej; Poggio, Tomaso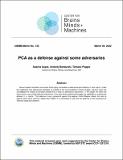
DownloadCBMM-Memo-135.pdf (2.575Mb)
Metadata
Show full item recordAbstract
Neural network classifiers are known to be highly vulnerable to adversarial perturbations in their inputs. Under the hypothesis that adversarial examples lie outside of the sub-manifold of natural images, previous work has investigated the impact of principal components in data on adversarial robustness. In this paper we show that there exists a very simple defense mechanism in the case where adversarial images are separable in a previously defined $(k,p)$ metric. This defense is very successful against the popular Carlini-Wagner attack, but less so against some other common attacks like FGSM. It is interesting to note that the defense is still successful for relatively large perturbations.
Date issued
2022-03-30Publisher
Center for Brains, Minds and Machines (CBMM)
Series/Report no.
CBMM Memo;135