Parsing Occluded People by Flexible Compositions
Author(s)
Chen, Xianjie; Yuille, Alan L.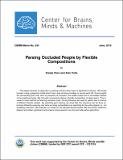
DownloadCBMM-Memo-034.pdf (5.535Mb)
Terms of use
Metadata
Show full item recordAbstract
This paper presents an approach to parsing humans when there is significant occlusion. We model humans using a graphical model which has a tree structure building on recent work [32, 6] and exploit the connectivity prior that, even in presence of occlusion, the visible nodes form a connected subtree of the graphical model. We call each connected subtree a flexible composition of object parts. This involves a novel method for learning occlusion cues. During inference we need to search over a mixture of different flexible models. By exploiting part sharing, we show that this inference can be done extremely efficiently requiring only twice as many computations as searching for the entire object (i.e., not modeling occlusion). We evaluate our model on the standard benchmarked “We Are Family" Stickmen dataset and obtain significant performance improvements over the best alternative algorithms.
Date issued
2015-06-01Publisher
Center for Brains, Minds and Machines (CBMM), arXiv
Citation
arXiv:1412.1526
Series/Report no.
CBMM Memo Series;034
Keywords
Occlusion, Inference, Machine Learning, Artificial Intelligence
Collections
The following license files are associated with this item: